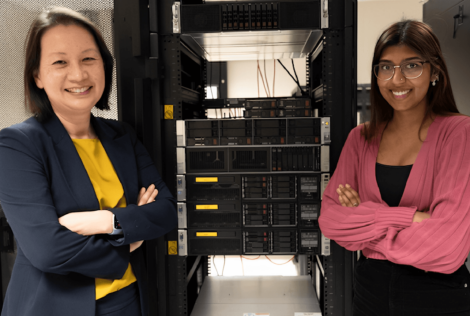
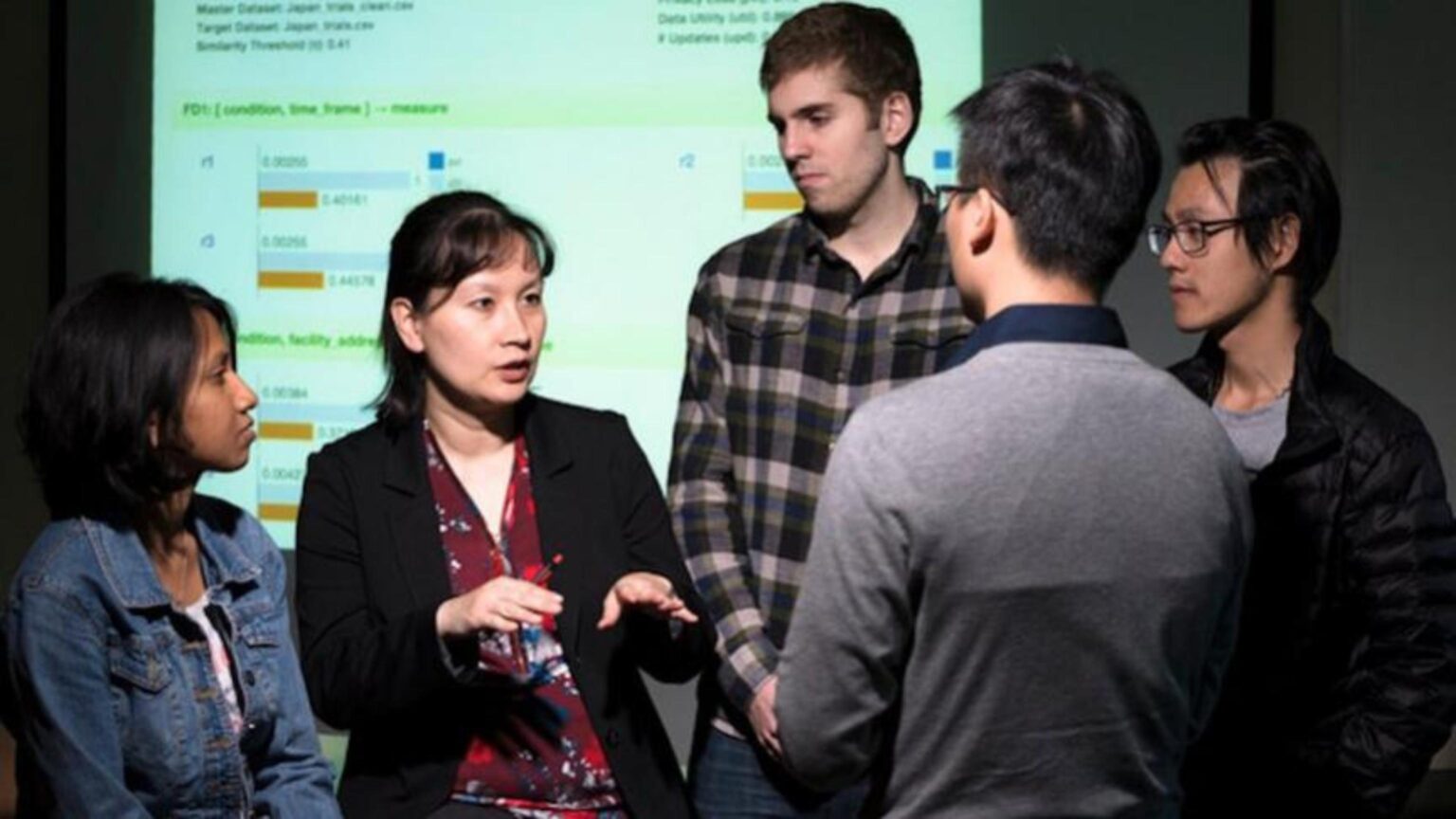
How is it that your financial institution, cell phone company or local cable provider knows everything about you from your postal code to your mother’s maiden name, but they can’t figure out how to correct extra charges on your monthly statement without bouncing you from one department to another, or putting you on hold for an interminable amount of time?
It’s an all-too-familiar scenario that underscores one of the challenges of big data that extends beyond volume, velocity and variety.
For Fei Chiang, the challenge is ensuring quality over quantity, focussing on the veracity and value of the data – whether or not it’s accurate, consistent and timely.
“Many companies simply aren’t sharing the data they’ve collected on their customers,” says Chiang, associate director of the MacDATA Research Institute. “If updates aren’t done across departments, then the department that knows the details about your service plan won’t know that you’ve moved, or perhaps changed the PIN on your account.”
For the computing and software professor, an easy solution is a central repository that keeps everyone in synch with the client’s most current data. However, this is not always practically feasible due to synchronization and concurrency issues.
Chiang and her research group focus on developing the techniques and tools that can repair, clean, validate and safeguard the massive datasets generated by telecommunications corporations, financial institutions, health care systems and industry. Their work ensures that high quality data is produced, which in turn ensures valuable insights and real-time decision making – right down to solving the issue with your cell phone provider in one short, efficient phone call.
Chiang’s Data Science Research Lab is involved in a number of projects, one of which involves a collaboration with IBM Canada. Her research team is tackling the challenge of developing efficient data cleaning algorithms that can be used in IBM’s product portfolio.
The software technology they’re developing would enable data cleaning tools that assess and predict a user’s intentions with the data. This would enable more customized software solutions that curate and clean the data according to the specific information a user is looking for.
“Take for example the sports industry – we would create a customized data profile view that could be used by the fan who wants the latest stats for their football pool, and another view for the sports station that requires in-depth analysis and predictions for each and every team in the NHL,” Chiang explains.
“Whether it’s a basketball scout, the wagering industry or a marketing firm trying to foresee the World Series MVP so they can lock up a promotions contract, we are building automated software tools to provide users with consistent and accurate data based on their specific information needs.”
The added bonus of working with IBM is that the prestigious global enterprise provides Chiang’s graduate students with entrée to the latest ‘real-world’ technologies and tools, plus access to modern data sets and the opportunity to solve data quality challenges.
Yu Huang, one of Chiang’s PhD students, has also had the opportunity to work with IBM’s Centre for Advanced Studies as an intern. The internship is every year for three years, connecting Huang with architects and software developers, where he gains not only leading edge technological knowledge, but industry relevant experience.
“It’s graduate students like Yu who are going to solve the next-generation challenges of Big Data, to harness its potential and power,” says Chiang.
“It isn’t just the vast amounts of data that make Big Data ‘big’. The real value lies in extracting the value from this data to produce accurate, high quality data, and to reap the information and insights this data provides.”